Distributions in R
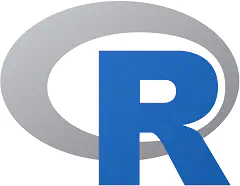
Introduction
This post contains some of my notes on the Bernoulli, geometric, Poisson, and exponential
distributions. I describe these distributions using R
’s functions and graphics. I
continue to work on this post to improve my understanding and gain a deeper insight into
probability distributions, particularly through the use of worked examples in R
.
Bernoulli distribution
Consider a simple experiment, which results in either a “success” or “failure”.
Let
be the event that the experiment results in a “success” and let be the event that it results in a “failure”.We define
as a discrete random variable having a Bernoulli distribution with parameter , where is a probability ( ). Formally, we denote , if has the probability mass function (pmf):
- A popular example of a Bernoulli distribution is a coin flip. We let
be a “heads” up result and be a “tails” up result and assume the coin is fair ( ). - We can simulate a coin flip in
R
using the functionrbinom(n, size, prob)
, wheren
is the number of observations,size
is the number of trials, andprob
is the probability of success ( ). The example produces the realization , a “tails” result.
set.seed(1200)
rbinom(n=1, size=1, p=0.5)
[1] 0
Binomial distribution
The Bernoulli distribution is not that useful because it models only a single event, for example, one coin flip. When we put many Bernoulli trials together, we get a binomial experiment.
A binomial experiment consists of
independent Bernoulli trials, where each trial result is a “success” or “failure”. The probability of “success” of each trial is and for “failure”. The trials are considered to be independent from one another, meaning that the results of any subset of trials do not affect the uncertainty of subsequent trials.A random variable
has a binomial distribution with parameter based on trials, and is denoted as . Let count the number of successes in independent trials, each with probability of success, where ( ).Then,
where are independent random variables all with a Bern( ) distribution. The pmf of the Bionomial distribution is:
- We can generate a realization of
using therbinom
function. Contining with the coin example, we flip a coinn=10
times and count the number of results that are “tails”.
x = rbinom(n=10, size=1, prob=0.5)
x
[1] 0 0 1 0 1 1 1 1 1 1
sum(x)
[1] 7
- The binomial distribution can be used to answer questions such as: what is the
probability that we observe 3 “heads” in 10 coin flips? We could
compute this probability using the function
dbinom(x, size, prob)
, wherex
is the number of successes andsize
is the number of trials. The probaility of seeing 3 “heads” in 10 coin flips, expressed as , is:
dbinom(x=3, size=10, prob=0.5)
[1] 0.1171875
- We can plot a binomial distribution for different values of
using thedbinom
function.
plot(0:20, dbinom(0:20, 20, p=0.5), type = "o",
col = "indianred1", xlab = "x (number of successes)", xlim=c(0, 40),
ylab = "Probability")
lines(0:40, dbinom(0:40, 40, p=0.4), type="o", col="skyblue3")
lines(0:20, dbinom(0:20, 20, p=0.6), type="o", col="darkseagreen3")
legend("topright", legend=c("n=20, p=0.5", "n=40, p=0.4", "n=20, p=0.6"),
bty="n", lty=1, col=c("indianred1", "skyblue3", "darkseagreen3"))

- As
gets large, the binomial distribution approaches the normal distribution.
Geometric distribution
In a geometric experiment, we ask how many Bernoulli trials must we run before we observe a “success”, or how many times must we flip a coin until we observe “heads”.
Again, we have
for the probability of success and is the number of independent trials before we observe a success.The pmf is of a Geometric distribution is:
In a game of darts, the probability of hitting the center area is
. What is , the probability that you hit the centre area on your th throw?We can use the
dgeom(x, prob)
function inR
to calculate the probability, wherex
is the number of trials (throws) andp
is the probability of success (hitting the centre area).
dgeom(8, 0.05)
[1] 0.03317102
#or
(1-0.05)^8 * 0.05
[1] 0.03317102
- We can vary
to obtain different geometric distributions.
plot(0:20, dgeom(0:20, p=0.5), type = "o",
col = "indianred1", xlab = "x (number of trials)",
xlim=c(0, 20), ylim=c(0, 1), ylab = "Probability")
lines(0:20, dgeom(0:20, p=0.2), type="o", col="skyblue3")
lines(0:20, dgeom(0:20, p=0.8), type="o", col="darkseagreen3")
legend("topright", legend=c("p=0.5", "p=0.2", "p=0.8"),
bty="n", lty=1, col=c("indianred1", "skyblue3", "darkseagreen3"))

- We can also ask what is the probability that it takes 8 or less trials (throws of the data) to hit the centre. This is expressed as:
We can use the pgeom
function to calculate this probability.
1 - (1-0.05)^(8+1)
[1] 0.3697506
# Or
pgeom(8, 0.05)
[1] 0.3697506
- We can also generate a realization of
usingrgeom(n, prob)
, where is the number of trials andprob
is the probability. With , the number of independent trials that must be run before observing a success is:
rgeom(1, 0.05)
[1] 12
- Of course, since
is a random variable that has a geometric distribution, we will observe a different realization for every trial.
x <- rgeom(10, 0.05)
x
[1] 11 27 6 7 42 3 13 4 34 67
In this example, we perform
Exponential distribution
The geometric distribution models how many times it takes for something (i.e., a success) to happen.
An exponential distribution is a continuous analog of the geometric distribution. The exponential distribution models how long it takes before a new customer enters the shop, how long before the next earthquake erupts, or how long it takes before the next piece of machinery breaks.
These questions involve an element of time, the time to wait until a given event occurs. (But not all exponential distributions involve a time component.) If the waiting time is unknown, then the random variable
has an exponential distribution with a single parameter .Since time is continuous, the exponential distribution has a probability density function (pdf):
The paramter
is a rate and represents the average number of events per interval. Let’s say that the average time for the next customer to arrive is minutes. Then the rate is one customer per 5 minutes or customers per minute.We can calculate the probability that a customer enters the store at 2 minutes using the
dexp
function.
(1/5) * exp(-(1/5 * 2))
[1] 0.134064
# Or
dexp(2, rate = 1/5)
[1] 0.134064
- We can visualize the exponential distribution using different values for
.
x <- seq(0, 5, by=0.05)
plot(x, dexp(x, rate = 1/5), type = "l",
col = "indianred1", xlab = "x",
xlim=c(0, 5), ylim=c(0, 1), ylab = "Probability density")
lines(x, dexp(x, rate=1/2), col="skyblue3")
lines(x, dexp(x, rate=2), col="darkseagreen3")
legend("topright",
bty="n", lty=1, col=c("indianred1", "skyblue3", "darkseagreen3"),
legend=c(expression(paste(lambda, " = 1/5")),
expression(paste(lambda, " = 1/2")),
expression(paste(lambda, " = 2"))))

Poisson distribution
A Poisson distribution is useful when we want to know the probability of observing
successes (or events) in a given time period or interval. For example, we may define as the number of cars that pass in an hour or the number of new HIV infections that we observe in the calendar year.The probability mass function of the Poisson distribution is given as:
is a random variable having the Poisson distribution, written as , where represents the average number of events per interval. This is the same definition of used in the exponential distribution.Consider a store that receives 10 customers between 16:00 and 17:00. Let
be the number of customers received in this interval, then has a Poisson distribution with .What is the probability of observing exactly 5 customers,
? We can calculate this probability inR
using thedpois(x, lambda)
function.
dpois(5, lambda = 10)
[1] 0.03783327
# or
(exp(-10) * (10^5))/factorial(5)
[1] 0.03783327
- We can vary
and to generate different Poisson distributions.
x = seq(0, 10)
plot(x, dpois(x, lambda=1.5), type = "o",
col = "indianred1", xlab = "x (number of events)",
xlim=c(0, 10), ylim=c(0, 0.7), ylab = "Probability")
lines(x, dpois(x, lambda=1.0), type="o", col="skyblue3")
lines(x, dpois(x, lambda=0.5), type="o", col="darkseagreen3")
legend("topright", bty="n", lty=1,
col=c("indianred1", "skyblue3", "darkseagreen3"),
legend=c(expression(paste(lambda, " = 1.5")),
expression(paste(lambda, " = 1.0")),
expression(paste(lambda, " = 0.5"))))

The Poisson model assumes that events are independent, that is, the occurrence of one event does not affect the probability that a second event will occur. It also assumes that the events are identically distributed, that the average rate of events is constant.
In addition, two events cannot occur at exactly the same instant. Instead, at each very small sub-interval exactly one event either occurs or does not occur. In this way, the Poisson distribution is also the limit of a binomial distribution, for which the probability of success for each trial equals
divided by the number of trials, as the number of trials approaches infinity.The exponential distribution is the probability distribution of time between events in a Poisson model. If the arrival times (of let’s say of customers) are Poisson with rate
, then the time between arrivals, known as the inter-arrival time, follows an exponential distribution, with as the average interval time. If times between arrivals are exponentially distributed with average inter-arrival time , then the arrivals follow the Poisson distribution with an average of arrivals per unit time.